Machine Learning Virus Detection: A Revolutionary Approach to Cybersecurity
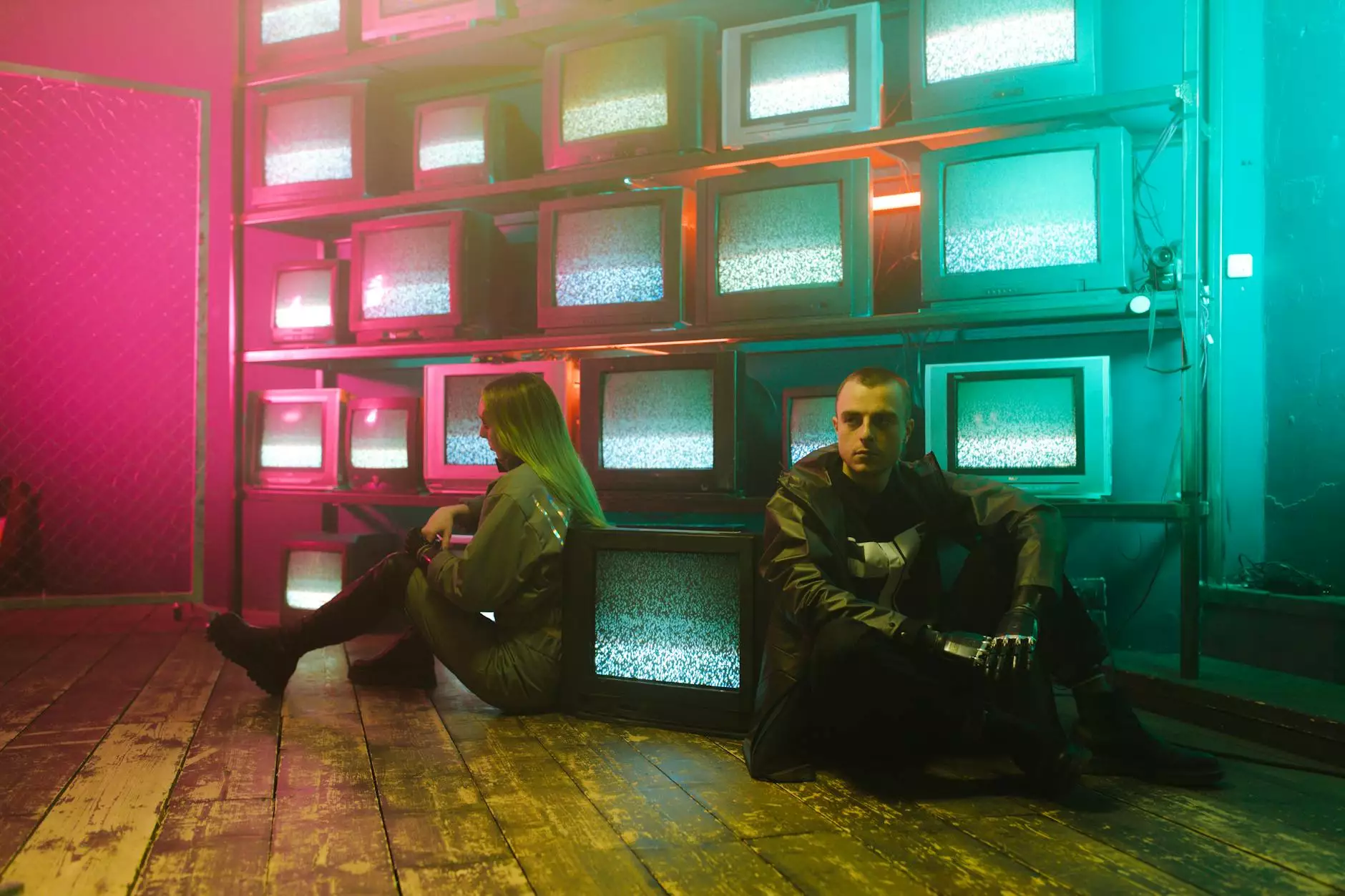
In today's digital landscape, businesses face an increasing number of threats from malicious software and cyberattacks. The need for robust security solutions has never been more urgent. Enter machine learning virus detection, a cutting-edge approach that harnesses the power of artificial intelligence to identify and combat threats in real-time.
Understanding the Basics of Machine Learning
To appreciate the significance of machine learning in virus detection, it is essential to understand the basic principles behind it. Machine learning is a subset of artificial intelligence that allows computer systems to learn from data, improve their performance over time, and make decisions without explicit programming.
How Does Machine Learning Virus Detection Work?
Machine learning algorithms analyze vast amounts of data to identify patterns and anomalies. In the context of virus detection, these algorithms are trained on datasets that include both benign and malicious software. Here's a breakdown of the process:
- Data Collection: Security experts gather extensive datasets of known viruses and benign files.
- Preprocessing: The data is cleaned and formatted to ensure consistency and quality.
- Model Training: Algorithms are trained using the preprocessed data to learn the characteristics of both malicious and legitimate files.
- Real-time Analysis: As new files are introduced into the system, the trained model evaluates their attributes and predicts their likelihood of being harmful.
- Continuous Learning: The machine learning model continues to improve by learning from new data and feedback, adapting to emerging threats.
The Advantages of Machine Learning Virus Detection
The transition from traditional virus detection methods to machine learning-based solutions offers several compelling benefits for businesses. Here are some of the key advantages:
- Proactive Threat Detection: Unlike traditional methods that rely on known signatures, machine learning can identify zero-day attacks and new malware variants, providing a layer of protection against unknown threats.
- Reduced False Positives: Machine learning algorithms are designed to learn from past experiences, which helps minimize false alarms and ensures that legitimate files are not mistakenly flagged.
- Scalability: As businesses grow, their IT infrastructure becomes more complex. Machine learning solutions can easily scale to handle increased data and user activity without a significant drop in performance.
- Speed and Efficiency: Machine learning algorithms can process large datasets much faster than human analysts, enabling quicker responses to potential threats.
- Cost-Effectiveness: By automating the detection process, businesses can reduce the manpower required for cybersecurity tasks, allowing IT teams to focus on strategic initiatives.
Integrating Machine Learning Virus Detection into Your IT Systems
For businesses looking to harness the power of machine learning virus detection, it is crucial to approach the integration process with a well-planned strategy. Here are some steps to consider:
Assessment and Planning
Begin by assessing your existing IT infrastructure and security posture. Identify vulnerabilities and areas that require improvement. Create a roadmap for implementing machine learning solutions tailored to your specific needs.
Choosing the Right Solution
There are numerous machine learning-based cybersecurity products available. When selecting a solution, consider factors such as:
- Reputation and reviews of the software
- Integration capabilities with your current systems
- Scalability to accommodate future growth
- Vendor support and training options
Implementation
Once you have chosen a solution, it is time to implement it across your IT systems. This may involve deploying software, configuring settings, and ensuring that all users are trained to utilize the new tools effectively.
Monitor and Optimize
After implementation, continuously monitor the performance of your machine learning virus detection system. Use analytics to assess its effectiveness and make necessary adjustments. Stay informed about the latest cybersecurity trends and updates from your solution provider.
The Role of Machine Learning in Future Cybersecurity
As technology continues to evolve, so do the tactics of cybercriminals. The ongoing development of machine learning virus detection promises to enhance cybersecurity significantly. The future may hold even more sophisticated algorithms that can respond to threats in real-time, leveraging predictive analysis to preemptively address vulnerabilities.
Potential Innovations
Future advancements in machine learning virus detection could include:
- Adversarial Machine Learning: An area where models learn to defend against adversarial attacks, thereby improving resilience.
- Automated Threat Hunting: Systems that autonomously seek out and neutralize threats before they can cause harm.
- Behavioral Analysis: Going beyond static signatures, future models may analyze user behavior and file interactions to detect anomalies.
Conclusion: Embracing Machine Learning for Enhanced Security
In an era where cyber threats are becoming increasingly sophisticated, businesses must adopt proactive measures to protect their data, assets, and reputation. Implementing machine learning virus detection can provide the advanced security needed to safeguard against emerging threats effectively.
At Spambrella, we understand the importance of cybersecurity and offer integrated IT services and computer repair solutions tailored to your business needs. Our expertise in security systems ensures that your company remains one step ahead in the fight against cybercrime. By leveraging machine learning technology, we can help you build a resilient firewall against potential threats.
Make the intelligent choice for your IT security. Invest in machine learning virus detection today and fortify your defenses against future cyber threats.